TechNews
Latest updates and insights on tech.
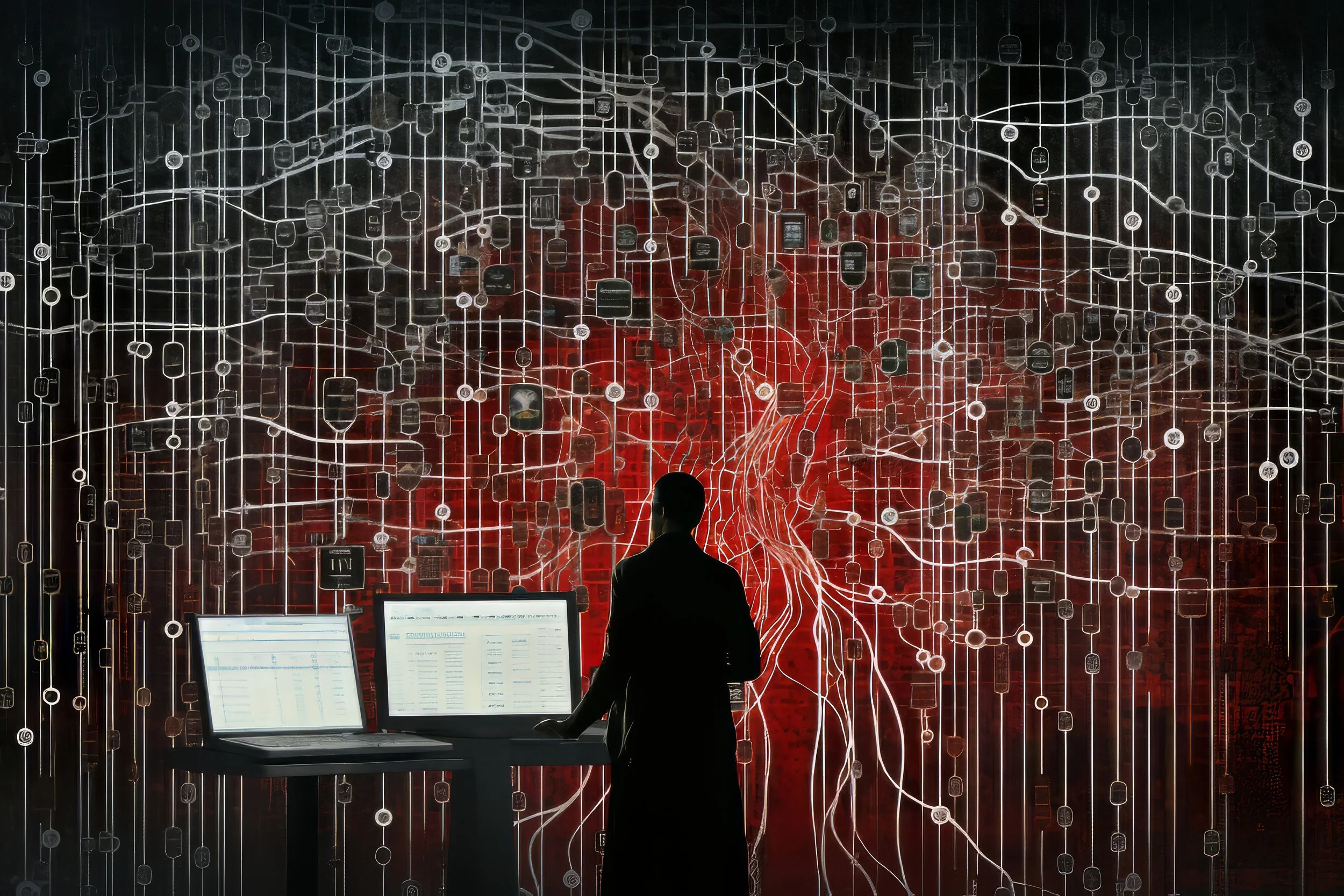
Exploring Neural Network Complexity
This article explores the complexity and potential of various neural network architectures. We delve into the fundamental building blocks of NNs, including perceptrons, activation functions, and learning algorithms. Subsequently, we explore different types of architectures, including feedforward networks, convolutional neural networks (CNNs), recurrent neural networks (RNNs), and deep learning architectures. We discuss the strengths and limitations of each architecture and showcase their diverse applications in various fields. Finally, the article examines emerging trends and future directions in neural network research,
Neural networks (NNs) are a powerful class of machine learning algorithms loosely inspired by the structure and function of the human brain. These networks consist of interconnected nodes, or artificial neurons, that process information and learn from data.
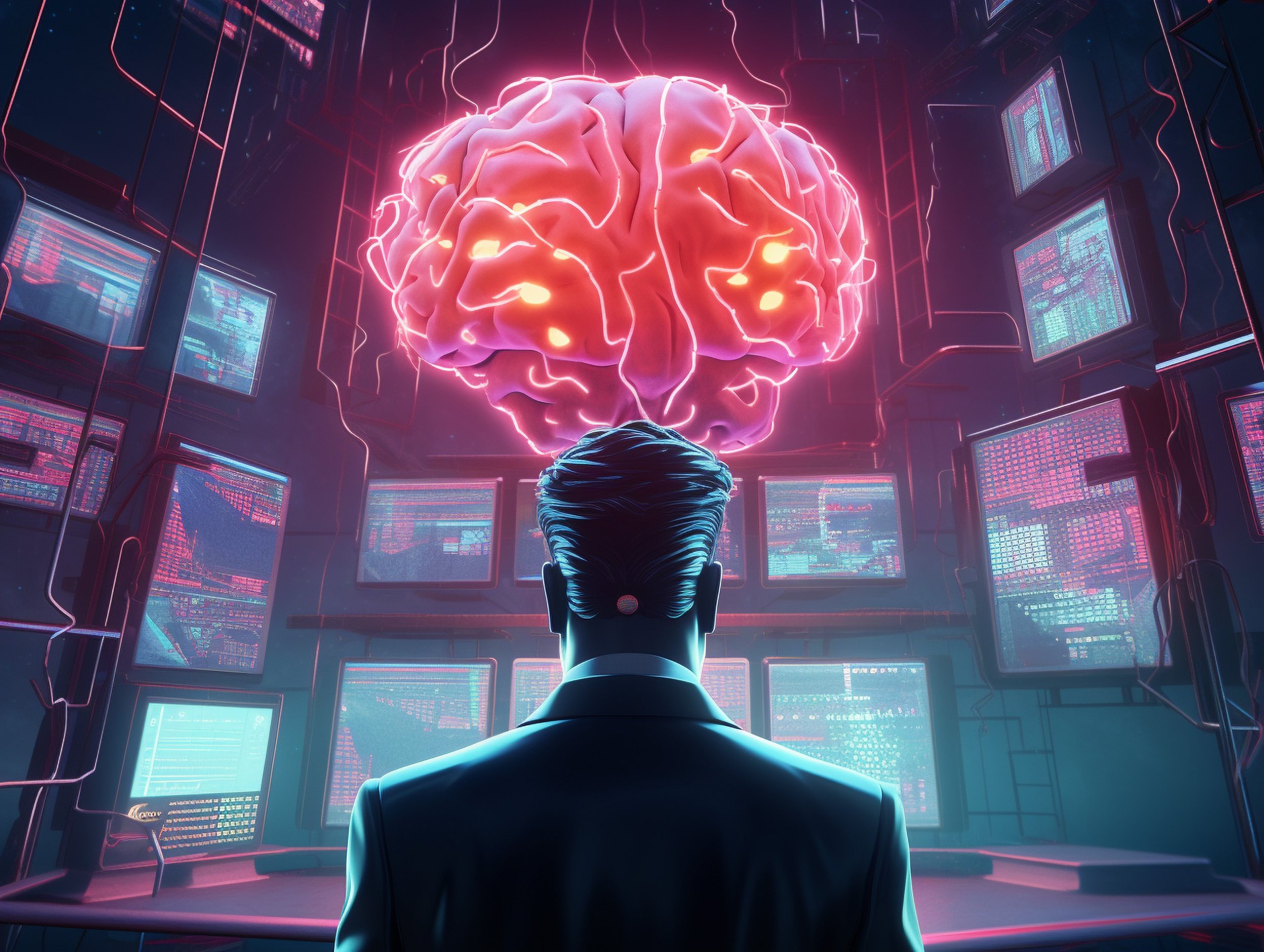
Generative Deep Learning
This article delves into the concept of generative models, exploring their core principles and the various techniques employed, with a particular focus on Generative Adversarial Networks (GANs). We discuss the applications of generative models in creating realistic images, sounds, and even text formats, highlighting their potential to revolutionize various creative industries and scientific research fields, Deep learning has revolutionized various fields by enabling machines to learn complex patterns from data. However, a significant limitation of traditional deep learning models lies in their focus on classification or prediction tasks. Generative models, a subfield of deep learning, address this gap by learning the underlying distribution of data and generating entirely new samples that resemble the training data.
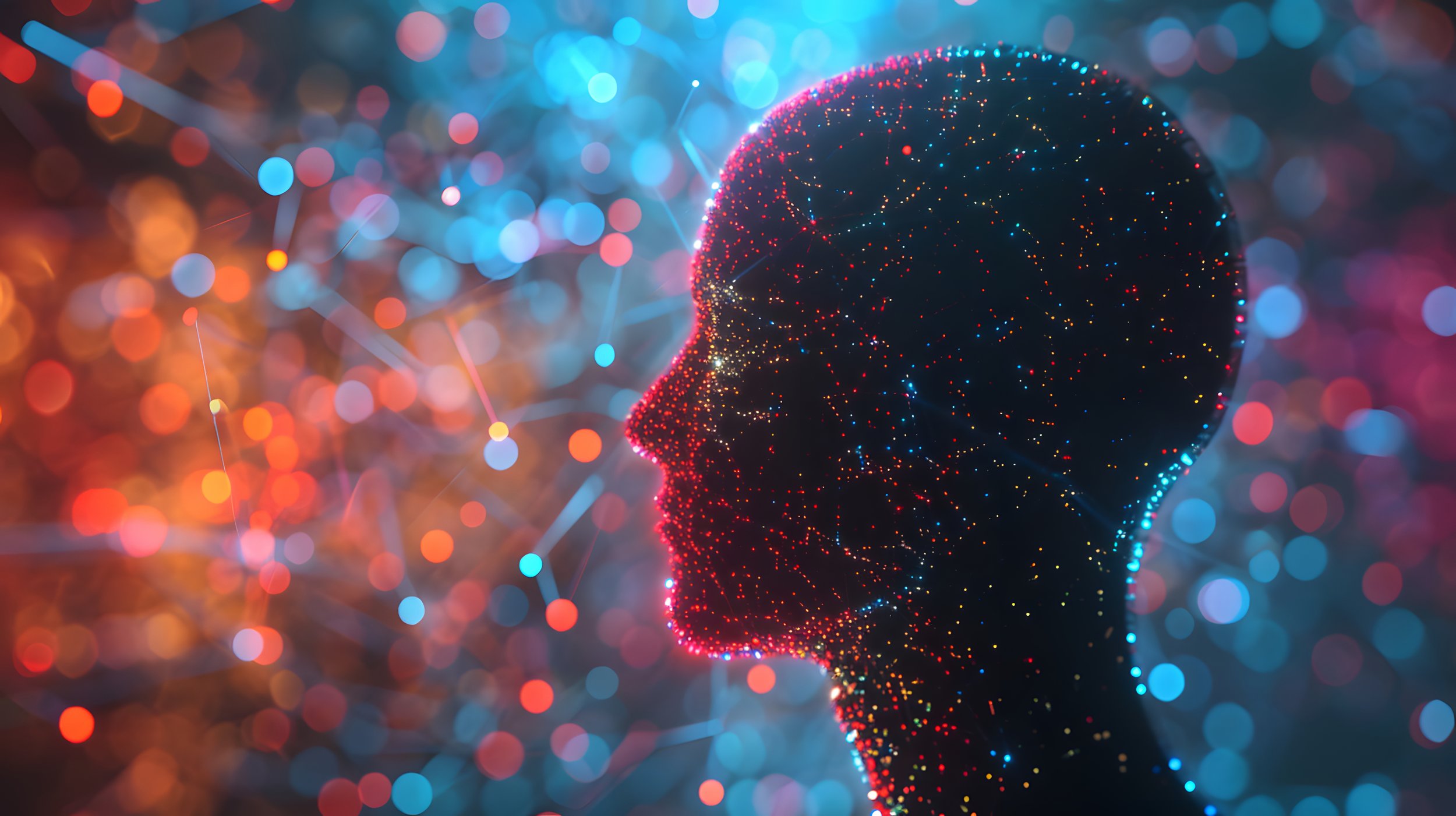
Generative Models: Data Creation
Deep learning has revolutionized various fields by enabling machines to learn complex patterns from data. However, a significant limitation of traditional deep learning models lies in their focus on classification or prediction tasks. Generative models, a subfield of deep learning, address this gap by learning the underlying distribution of data and generating entirely new samples that resemble the training data. This article delves into the concept of generative models, exploring their core principles and the various techniques employed, with a particular focus on Generative Adversarial Networks (GANs). We discuss the applications of generative models in creating realistic images, sounds, and even text formats, highlighting their potential to revolutionize various creative industries and scientific research fields.